London Town
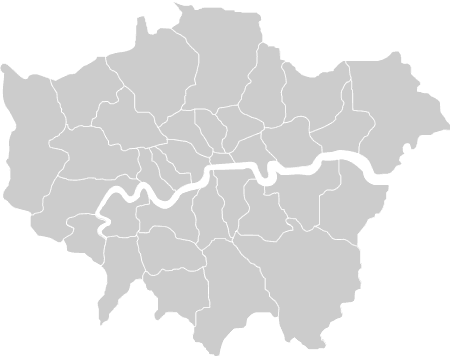
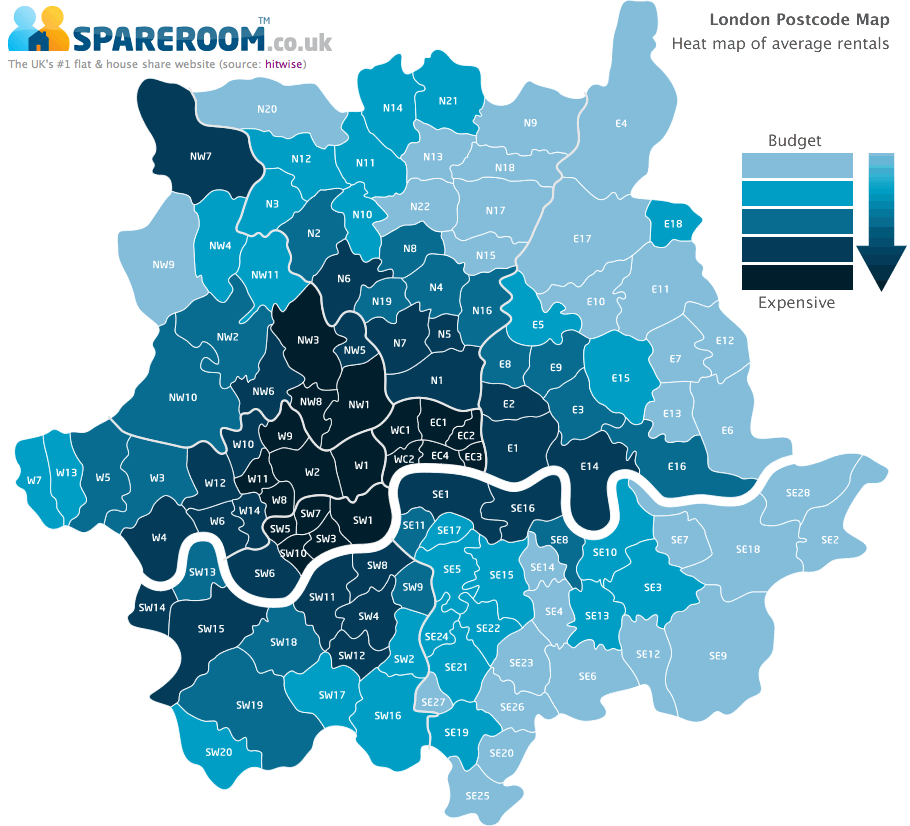
Check out London post codes…
Check out London post codes…
Why POC-D Training …
Note : This course is built on top of the “Real World Spark 2 – Jupyter Python Spark Core – Toyin Akin” course
Jupyter Notebook is a system similar to Mathematica that allows you to create “executable documents”. Notebooks integrate formatted text (Markdown), executable code (Python), mathematical formulas (LaTeX), and graphics and visualizations (matplotlib) into a single document that captures the flow of an exploration and can be exported as a formatted report or an executable script.,
The Jupyter Notebook is a web application that allows you to create and share documents that contain live code, equations, visualizations and explanatory text. Uses include: data cleaning and transformation, numerical simulation, statistical modeling, machine learning and much more.
Big data integration
Leverage big data tools, such as Apache Spark, from Python
The Jupyter Notebook is based on a set of open standards for interactive computing. Think HTML and CSS for interactive computing on the web. These open standards can be leveraged by third party developers to build customized applications with embedded interactive computing.
Spark Monitoring and Instrumentation
While creating RDDs, performing transformations and executing actions, you will be working heavily within the monitoring view of the Web UI.
Every SparkContext launches a web UI, by default on port 4040, that displays useful information about the application. This includes:
A list of scheduler stages and tasks A summary of RDD sizes and memory usage Environmental information. Information about the running executors
Why Apache Spark …
Apache Spark run programs up to 100x faster than Hadoop MapReduce in memory, or 10x faster on disk. Apache Spark has an advanced DAG execution engine that supports cyclic data flow and in-memory computing. Apache Spark offers over 80 high-level operators that make it easy to build parallel apps. And you can use it interactively from the Scala, Python and R shells. Apache Spark can combine SQL, streaming, and complex analytics.
Apache Spark powers a stack of libraries including SQL and DataFrames, MLlib for machine learning, GraphX, and Spark Streaming. You can combine these libraries seamlessly in the same application.
Note : This course is built on top of the “Real World Spark 2 – Interactive Python pyspark Core – Toyin Akin” course
Spark’s python shell provides a simple way to learn the API, as well as a powerful tool to analyze data interactively. It is available in Python. Start it by running the following anywhere within a bash terminal within the built Virtual Machine
pyspark
Spark’s primary abstraction is a distributed collection of items called a Resilient Distributed Dataset (RDD). RDDs can be created from collections, Hadoop InputFormats (such as HDFS files) or by transforming other RDDs
Spark Monitoring and Instrumentation
While creating RDDs, performing transformations and executing actions, you will be working heavily within the monitoring view of the Web UI.
Every SparkContext launches a web UI, by default on port 4040, that displays useful information about the application. This includes:
A list of scheduler stages and tasks A summary of RDD sizes and memory usage Environmental information. Information about the running executors
Why Apache Spark …
Apache Spark run programs up to 100x faster than Hadoop MapReduce in memory, or 10x faster on disk. Apache Spark has an advanced DAG execution engine that supports cyclic data flow and in-memory computing. Apache Spark offers over 80 high-level operators that make it easy to build parallel apps. And you can use it interactively from the Scala, Python and R shells. Apache Spark can combine SQL, streaming, and complex analytics.
Apache Spark powers a stack of libraries including SQL and DataFrames, MLlib for machine learning, GraphX, and Spark Streaming. You can combine these libraries seamlessly in the same application.
Note : This course is built on top of the “Real World Spark 2 – Interactive Scala spark-shell Core – Toyin Akin” course
Spark’s shell provides a simple way to learn the API, as well as a powerful tool to analyze data interactively. It is available in Scala (which runs on the Java VM and is thus a good way to use existing Java libraries). Start it by running the following anywhere within a bash terminal within the built Virtual Machine
spark-shell
Spark’s primary abstraction is a distributed collection of items called a Resilient Distributed Dataset (RDD). RDDs can be created from collections, Hadoop InputFormats (such as HDFS files) or by transforming other RDDs
Spark Monitoring and Instrumentation
While creating RDDs, performing transformations and executing actions, you will be working heavily within the monitoring view of the Web UI.
Every SparkContext launches a web UI, by default on port 4040, that displays useful information about the application. This includes:
A list of scheduler stages and tasks A summary of RDD sizes and memory usage Environmental information. Information about the running executors
Why Apache Spark …
Apache Spark run programs up to 100x faster than Hadoop MapReduce in memory, or 10x faster on disk. Apache Spark has an advanced DAG execution engine that supports cyclic data flow and in-memory computing. Apache Spark offers over 80 high-level operators that make it easy to build parallel apps. And you can use it interactively from the Scala, Python and R shells. Apache Spark can combine SQL, streaming, and complex analytics.
Apache Spark powers a stack of libraries including SQL and DataFrames, MLlib for machine learning, GraphX, and Spark Streaming. You can combine these libraries seamlessly in the same application.